ISTQB Certified Tester AI Testing
The ISTQB® Certified AI Testing (CT-AI) certification deepens your understanding of artificial intelligence and machine learning, with a focus on ...
testing AI-based systems and leveraging AI in testing. Gain expertise in evaluating the unique challenges and methodologies involved in AI testing. Learn to effectively use AI tools and techniques to enhance the testing process and ensure the quality of AI-driven solutions.
Show more
Instructor
AcumenITTrainingInc
0
0 reviews
- Description
- Reviews
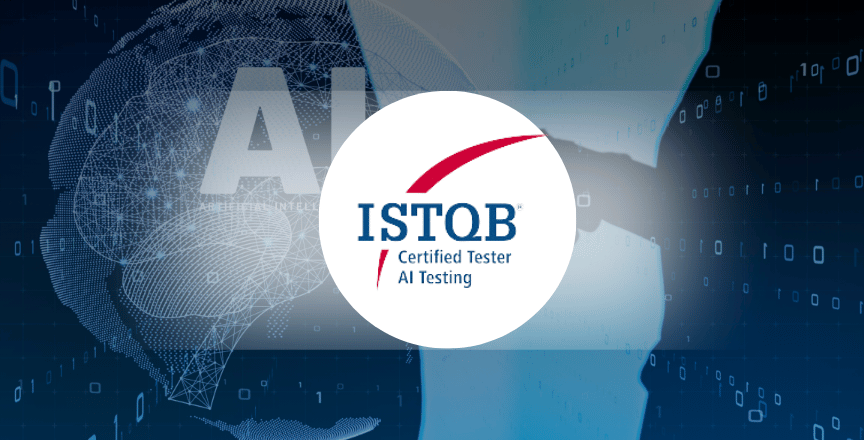
ISTQB CERTIFIED TESTER AI TESTING (CT-AI)
COURSE DESCRIPTION
The ISTQB® AI Testing (CT-AI) certification extends understanding of artificial intelligence and/or deep (machine) learning, most specifically testing AI-based systems and using AI in testing.
COURSE OBJECTIVES
- Understand the current state and expected trends of AI
- Experience the implementation and testing of a ML model and recognize where testers can best influence its quality
- Understand the challenges associated with testing AI-Based systems, such as their self-learning capabilities, bias, ethics, complexity, non-determinism, transparency and explainability
- Contribute to the test strategy for an AI-Based system
- Design and execute test cases for AI-based systems
- Recognize the special requirements for the test infrastructure to support the testing of AI-based systems
- Understand how AI can be used to support software testing
PREREQUISITES
To gain this certification, candidates must hold the Certified Tester Foundation Level certificate.
COURSE OUTLINE
- Introduction to AI
- Definition of AI and AI Effect
- Narrow, General and Super AI
- AI-Based and Conventional Systems
- AI Technologies
- AI Development Frameworks
- Hardware for AI-Based Systems
- AI as a Service (AIaaS)
- Contracts for AI as a Service
- AIaaS Examples
- Pre-Trained Models
- Introduction to Pre-Trained Models
- Transfer Learning
- Risks of using Pre-Trained Models and Transfer Learning
- Standards, Regulations and AI
- Quality Characteristics for AI-Based Systems
- Flexibility and Adaptability
- Autonomy
- Evolution
- Bias
- Ethics
- Side Effects and Reward Hacking
- Transparency, Interpretability and Explainability
- Safety and AI
- Machine Learning (ML) – Overview
- Forms of ML
- Supervised Learning
- Unsupervised Learning
- Reinforcement Learning
- Workflow
- Selecting Form of ML
- Factors Involved in ML Algorithm Selection
- Overfitting and Underfitting
- Overfitting
- Underfitting
- Hands-Exercise: Demonstrate Overfitting and Underfitting
- Forms of ML
- ML – Data
- Data Preparation as Part of the ML Workflow
- Challenges in Data Preparation
- Hands-On Exercise: Data Preparation for ML
- Training, Validation and Test Datasets in the ML Workflow
- Hands-On Exercise: Identify Training and Test Data and Create an ML Model
- Dataset Quality Issues
- Data Quality and its Effect on the ML Model
- Data Labelling for Supervised Learning
- Approaches to Data Labelling
- Mislabeled Data in Datasets
- Data Preparation as Part of the ML Workflow
- ML Functional Performance Metrics
- Confusion Matrix
- Additional ML Functional Performance Metrics for Classification, Regression and Clustering
- Limitations of ML Functional Performance Metrics
- Selecting ML Functional Performance Metrics
- Hands-On Exercise: Evaluate the Created ML Model
- Benchmark Suites for ML
- ML – Neural Networks and Testing
- Neural Networks
- Hands-On Exercise: Implement a Simple Perceptron
- Coverage Measures for Neural Networks
- Neural Networks
- Testing AI-Based Systems Overview
- Specification of AI-Based Systems
- Test Levels for AI-Based Systems
- Input Data Testing
- ML Model Testing
- Component Testing
- Component Integration Testing
- System Testing
- Acceptance Testing
- Test Data for Testing AI-based Systems
- Testing for Automation Bias in AI-Based Systems
- Documenting an AI Component
- Testing for Concept Drift
- Selecting a Test Approach for an ML System
- Testing AI-Specific Quality Characteristics
- Challenges Testing Self-Learning Systems
- Testing Autonomous AI-Based Systems
- Testing for Algorithmic, Sample and Inappropriate Bias
- Challenges Testing Probabilistic and Non-Deterministic AI-Based Systems
- Challenges Testing Complex AI-Based Systems
- Testing the Transparency, Interpretability and Explainability of AI-Based Systems
-
- Hands-On Exercise: Model Explainability
- Test Oracles for AI-Based Systems
- Test Objectives and Acceptance Criteria
- Methods and Techniques for the Testing of AI-Based Systems
- Adversarial Attacks and Data Poisoning
- Adversarial Attacks
- Data Poisoning
- Pairwise Testing
- Hands-On Exercise: Pairwise Testing
- Back-to-Back Testing
- A/B Testing
- Metamorphic Testing (MT)
- Hands-On Exercise: Metamorphic Testing
- Experience-Based Testing of AI-Based Systems
- Hands-On Exercise: Exploratory Testing and Exploratory Data Analysis (EDA)
- Selecting Test Techniques for AI-Based Systems
- Adversarial Attacks and Data Poisoning
- Test Environments for AI-Based Systems
- Test Environments for AI-Based Systems
- Virtual Test Environments for Testing AI-Based Systems
- Using AI for Testing
- AI Technologies for Testing
- Hands-On Exercise: The Use of AI in Testing
- Using AI to Analyze Reported Defects
- Using AI for Test Case Generation
- Using AI for the Optimization of Regression Test Suites
- Using AI for Defect Prediction
-
- Hands-On Exercise: Build a Defect Prediction System
- Using AI for Testing User Interfaces
- Using AI to Test Through the Graphical User Interface (GUI)
- Using AI to Test the GUI
- AI Technologies for Testing
Please contact us for the full course outline, schedules and for booking a private class.
Please, login to leave a review
Related courses
Share
Course details
Duration
4 Days
Popular courses
Popular Courses
Archive
Working hours
Monday | 9:00 am - 6.00 pm |
Tuesday | 9:00 am - 6.00 pm |
Wednesday | 9:00 am - 6.00 pm |
Thursday | 9:00 am - 6.00 pm |
Friday | 9:00 am - 6.00 pm |
Saturday | Closed |
Sunday | Closed |